In the complex world of batteries, the State of Health (SOH) is a crucial parameter determining a battery’s overall well-being and remaining useful life. Equally important is the State of Charge (SOC) estimation, especially when it comes to SOC estimation for dry goods batteries, where accurate monitoring can significantly influence battery performance and longevity. Advancements in precise SOC estimation technology have made it easier to optimize the state of charge, ensuring maximum efficiency.
Accurate estimation of both SOH and SOC is essential for maximizing battery performance, refining charging strategies, and ensuring long-term reliability. This article explores various methods for estimating SOH, while also addressing how battery state of charge monitoring plays a pivotal role in this process. We will highlight the strengths, limitations, and emerging trends in SOC measurement for dry batteries and SOH that promise to revolutionize our understanding of battery health.
Cycle Counting: Traditional Approach
Cycle counting is a traditional method for estimating SOH. It assumes that a battery’s capacity gradually degrades with each charging and discharging cycle. This method provides a straightforward estimate of SOH by keeping track of the total number of cycles. While relatively simple to implement, it also serves as an initial method in SOC estimation techniques.
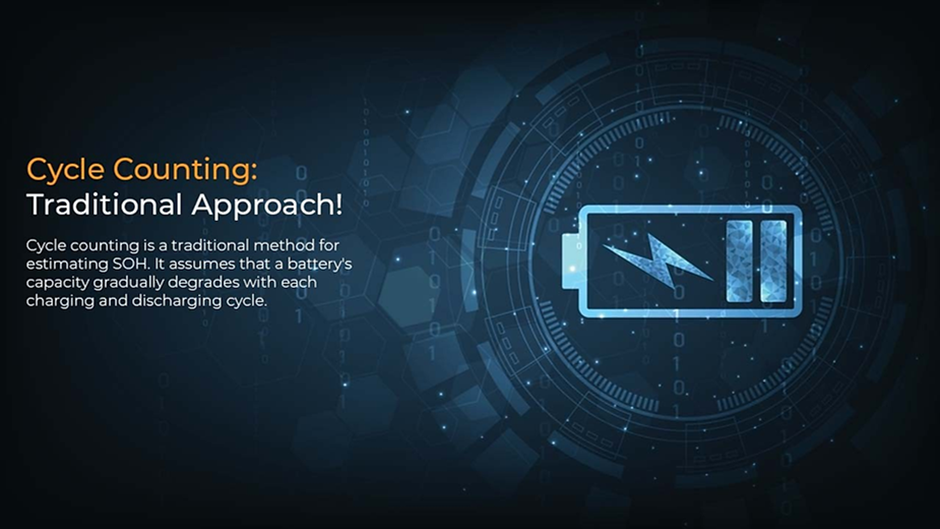
However, cycle counting has limitations. It oversimplifies the complex factors influencing battery degradation, such as depth of discharge (DOD), temperature variations, and charging patterns. This simplistic approach may lead to inaccurate estimations, especially with modern usage patterns involving partial charging and discharging cycles. As such, advancements in SOC estimation are critical in complementing this traditional method for better accuracy.
Charging Capacity Analysis: Precision Meets Complexity
Charging capacity analysis takes a more dynamic approach to SOH estimation by analyzing the actual charging capacity of the battery. This method compares the energy stored during a charging cycle with the original capacity, providing a more accurate assessment of both battery health and SOC algorithms for batteries.
While charging capacity analysis considers various factors impacting battery performance, it comes with its challenges. Precise measurement often requires sophisticated equipment or accurate SOC estimation methods, increasing implementation costs and complexity. Moreover, its accuracy is highest when the battery is charged from a low state of charge (SOC) to a fully charged state, potentially underestimating capacity decline with frequent charging from higher SOC levels.
Combining Cycle Counting with Charging Capacity Analysis
Recognizing the limitations of individual methods, a contemporary trend in SOH estimation involves combining cycle counting with charging capacity analysis. This synergistic approach aims to comprehensively evaluate battery degradation, considering both cumulative cycles and dynamic variations in charging behaviors and environmental conditions. Real-time SOC estimation for batteries and innovations in battery SOC tracking is integral to this comprehensive evaluation.
Emerging Trends in SOH and SOC Estimation
- Machine Learning (ML): Precision and Dynamism: Incorporating machine learning algorithms trained on extensive battery data has emerged as a game-changer. ML goes beyond traditional methods, considering factors beyond cycle count and charging capacity. This approach enables more accurate and dynamic predictions of SOH and SOC prediction advancements.
- Electrochemical Impedance Spectroscopy (EIS): Unveiling Internal Dynamics: EIS, a technique analyzing the battery’s internal resistance, offers insights into its health and facilitates early detection of potential degradation issues. It’s a key element in improving battery state of charge monitoring and overall, SOC improvement for dry goods batteries.
- Open-Circuit Voltage (OCV) Analysis: Monitoring the Unseen: OCV analysis involves monitoring the battery’s open-circuit voltage during charging and discharging cycles, providing valuable information about its health and remaining capacity. This method adds another layer of precision to the SOH estimation process and can further support the battery management system SOC.
Conclusion: Navigating Towards Precision in SOH and SOC Estimation
In the ever-evolving landscape of battery technology, precise estimation of both the State of Health and State of Charge is crucial. By understanding the strengths and limitations of conventional methods like cycle counting and charging capacity analysis, coupled with embracing emerging techniques such as machine learning, EIS, and OCV analysis, we pave the way for a comprehensive understanding of battery health and charge.
Ongoing advancements in SOC estimation and battery SOC prediction advancements hold immense promise for enhancing the accuracy and reliability of SOH and SOC estimation, ultimately optimizing battery performance, lifespan, and sustainability in the long run.